SaaSHub helps you find the best software and product alternatives Learn more →
Kedro Alternatives
Similar projects and alternatives to Kedro
-
WorkOS
The modern identity platform for B2B SaaS. The APIs are flexible and easy-to-use, supporting authentication, user identity, and complex enterprise features like SSO and SCIM provisioning.
-
openpilot
openpilot is an open source driver assistance system. openpilot performs the functions of Automated Lane Centering and Adaptive Cruise Control for 250+ supported car makes and models.
-
PRAW
PRAW, an acronym for "Python Reddit API Wrapper", is a python package that allows for simple access to Reddit's API.
-
InfluxDB
Power Real-Time Data Analytics at Scale. Get real-time insights from all types of time series data with InfluxDB. Ingest, query, and analyze billions of data points in real-time with unbounded cardinality.
-
cookiecutter-data-science
A logical, reasonably standardized, but flexible project structure for doing and sharing data science work.
-
DurableTask
Durable Task Framework allows users to write long running persistent workflows in C# using the async/await capabilities.
-
SaaSHub
SaaSHub - Software Alternatives and Reviews. SaaSHub helps you find the best software and product alternatives
Kedro reviews and mentions
-
Nextflow: Data-Driven Computational Pipelines
Interesting, thanks for sharing. I'll definitely take a look, although at this point I am so comfortable with Snakemake, it is a bit hard to imagine what would convince me to move to another tool. But I like the idea of composable pipelines: I am building a tool (too early to share) that would allow to lay Snakemake pipelines on top of each other using semi-automatic data annotations similar to how it is done in kedro (https://github.com/kedro-org/kedro).
-
A Polars exploration into Kedro
# pyproject.toml [project] dependencies = [ "kedro @ git+https://github.com/kedro-org/kedro@3ea7231", "kedro-datasets[pandas.CSVDataSet,polars.CSVDataSet] @ git+https://github.com/kedro-org/kedro-plugins@3b42fae#subdirectory=kedro-datasets", ]
-
What are some open-source ML pipeline managers that are easy to use?
So there's 2 sides to pipeline management: the actual definition of the pipelines (in code) and how/when/where you run them. Some tools like prefect or airflow do both of them at once, but for the actual pipeline definition I'm a fan of https://kedro.org. You can then use most available orchestrators to run those pipelines on whatever schedule and architecture you want.
-
How do data scientists combine Kedro and Databricks?
We have set up a milestone on GitHub so you can check in on our progress and contribute if you want to. To suggest features to us, report bugs, or just see what we're working on right now, visit the Kedro projects on GitHub.
-
How do you organize yourself during projects?
you could use a project framework like kedro to force you to be more disciplined about how you structure your projects. I'd also recommend checking out this book: Edna Ridge - Guerrilla Analytics: A Practical Approach to Working with Data
-
Futuristic documentation systems in Python, part 1: aiming for more
Recently I started a position as Developer Advocate for Kedro, an opinionated data science framework, and one of the things we're doing is exploring what are the best open source tools we can use to create our documentation.
-
Python projects with best practices on Github?
You can also check out Kedro, it’s like the Flask for data science projects and helps apply clean code principles to data science code.
- Data Science/ Analyst Zertifikate für den Job Markt?
- What are examples of well-organized data science project that I can see on Github?
-
Dabbling with Dagster vs. Airflow
An often overlooked framework used by NASA among others is Kedro https://github.com/kedro-org/kedro. Kedro is probably the simplest set of abstractions for building pipelines but it doesn't attempt to kill Airflow. It even has an Airflow plugin that allows it to be used as a DSL for building Airflow pipelines or plug into whichever production orchestration system is needed.
-
A note from our sponsor - SaaSHub
www.saashub.com | 27 Apr 2024
Stats
kedro-org/kedro is an open source project licensed under Apache License 2.0 which is an OSI approved license.
The primary programming language of Kedro is Python.
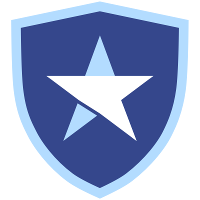
Sponsored