The APIs are flexible and easy-to-use, supporting authentication, user identity, and complex enterprise features like SSO and SCIM provisioning. Learn more →
Bootcamp Alternatives
Similar projects and alternatives to bootcamp
-
txtai
💡 All-in-one open-source embeddings database for semantic search, LLM orchestration and language model workflows
-
WorkOS
The modern identity platform for B2B SaaS. The APIs are flexible and easy-to-use, supporting authentication, user identity, and complex enterprise features like SSO and SCIM provisioning.
-
FLiPStackWeekly
FLaNK AI Weekly covering Apache NiFi, Apache Flink, Apache Kafka, Apache Spark, Apache Iceberg, Apache Ozone, Apache Pulsar, and more...
-
InfluxDB
Power Real-Time Data Analytics at Scale. Get real-time insights from all types of time series data with InfluxDB. Ingest, query, and analyze billions of data points in real-time with unbounded cardinality.
-
vosk-api
Offline speech recognition API for Android, iOS, Raspberry Pi and servers with Python, Java, C# and Node
-
annoy
Approximate Nearest Neighbors in C++/Python optimized for memory usage and loading/saving to disk
-
nn
🧑🏫 60 Implementations/tutorials of deep learning papers with side-by-side notes 📝; including transformers (original, xl, switch, feedback, vit, ...), optimizers (adam, adabelief, sophia, ...), gans(cyclegan, stylegan2, ...), 🎮 reinforcement learning (ppo, dqn), capsnet, distillation, ... 🧠
-
graphql-ws
Coherent, zero-dependency, lazy, simple, GraphQL over WebSocket Protocol compliant server and client.
-
imodels
Interpretable ML package 🔍 for concise, transparent, and accurate predictive modeling (sklearn-compatible).
-
es-clip-image-search
Sample implementation of natural language image search with OpenAI's CLIP and Elasticsearch or Opensearch.
-
SaaSHub
SaaSHub - Software Alternatives and Reviews. SaaSHub helps you find the best software and product alternatives
bootcamp reviews and mentions
- FLaNK AI - 01 April 2024
- FLaNK Stack Weekly 22 January 2024
-
Milvus Adventures Jan 5, 2023
Metadata Filtering with Zilliz Cloud Pipelines This tutorial discuss scalar or metadata filtering and how you can perform metadata filtering in Zilliz Cloud. This blog continues on the previous blog on Getting started with RAG in just 5 minutes. You can find its code in this notebook and scroll down to Cell #27.
-
Build a search engine, not a vector DB
Partially agree.
Vector DBs are critical components in retrieval systems. What most applications need are retrieval systems, rather than building blocks of retrieval systems. That doesn't mean the building blocks are not important.
As someone working on vector DB, I find many users struggling in building their own retrieval systems with building blocks such as embedding service (openai,cohere), logic orchestration framework (langchain/llamaindex) and vector databases, some even with reranker models. Putting them together is not as easy as it looks. A fairly changeling system work. Letting alone quality tuning and devops.
The struggle is no surprise to me, as tech companies who are experts on this (google,meta) all have dedicated teams working on retrieval system alone, making tons of optimizations and develop a whole feedback loop of evaluating and improving the quality. Most developers don't get access to such resource.
No one size fits all. I think there shall exist a service that democratize AI-powered retrieval, in simple words the know-how of using embedding+vectordb and a bunch of tricks to achieve SOTA retrieval quality.
With this idea I built a Retrieval-as-a-service solution, and here is its demo:
https://github.com/milvus-io/bootcamp/blob/master/bootcamp/R...
Curious to learn your thoughts.
-
Vector Database in a Jupyter Notebook
Although it's common to use vector databases in conjunction with LLMs, I like to talk about vector databases in the context of unstructured data, i.e. any data that you can vectorize with (or without) an ML model. Yes, this includes text, but it also includes things like visual data, molecular structures, and geospatial data.
For folks who want to learn a bit more, there are examples of vector database use cases beyond semantic text search in our bootcamp: https://github.com/milvus-io/bootcamp
-
Beginner-ish resources for choosing a vector database?
Easy to get started: Here are some tutorials for Milvus in a Jupyter Notebook that I wrote - reverse image search, semantic text search
-
Semantic Similarity Search
I think you can just store your vector embeddings in the vector store somewhere and then query with your second document. I created a short tutorial on this that shows how to get the top 2 vector embeddings from a text query
-
[D] Looking for open source projects to contribute
For more beginner tasks associated with the Milvus vector database, you can contribute to the Bootcamp project( https://github.com/milvus-io/bootcamp), where we build a lot of data-driven solutions using ML and Milvus vector database, including reverse image search, recommender systems, etc.
-
I built an image similarity search system... Suggestions needed: what are some fun image datasets or scenarios I can use with this? :)
Source code here: https://github.com/milvus-io/bootcamp/tree/master/solutions/reverse_image_search
- Faiss: Facebook's open source vector search library
-
A note from our sponsor - WorkOS
workos.com | 27 Apr 2024
Stats
milvus-io/bootcamp is an open source project licensed under Apache License 2.0 which is an OSI approved license.
The primary programming language of bootcamp is HTML.
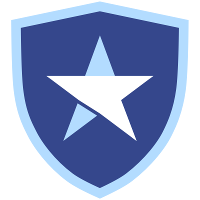
Sponsored