SaaSHub helps you find the best software and product alternatives Learn more →
Yolor Alternatives
Similar projects and alternatives to yolor
-
darknet
YOLOv4 / Scaled-YOLOv4 / YOLO - Neural Networks for Object Detection (Windows and Linux version of Darknet ) (by AlexeyAB)
-
InfluxDB
Power Real-Time Data Analytics at Scale. Get real-time insights from all types of time series data with InfluxDB. Ingest, query, and analyze billions of data points in real-time with unbounded cardinality.
-
yolov7
Implementation of paper - YOLOv7: Trainable bag-of-freebies sets new state-of-the-art for real-time object detectors
-
YOLOX
YOLOX is a high-performance anchor-free YOLO, exceeding yolov3~v5 with MegEngine, ONNX, TensorRT, ncnn, and OpenVINO supported. Documentation: https://yolox.readthedocs.io/
-
SaaSHub
SaaSHub - Software Alternatives and Reviews. SaaSHub helps you find the best software and product alternatives
-
tensorflow-yolov4-tflite
YOLOv4, YOLOv4-tiny, YOLOv3, YOLOv3-tiny Implemented in Tensorflow 2.3.1, Android. Convert YOLO v4 .weights tensorflow, tensorrt and tflite (by haroonshakeel)
-
MMdnn
MMdnn is a set of tools to help users inter-operate among different deep learning frameworks. E.g. model conversion and visualization. Convert models between Caffe, Keras, MXNet, Tensorflow, CNTK, PyTorch Onnx and CoreML.
-
PPYOLOE_pytorch
An unofficial implementation of Pytorch version PP-YOLOE,based on Megvii YOLOX training code.
-
yolo_series_deepsort_pytorch
Deepsort with yolo series. This project support the existing yolo detection model algorithm (YOLOV8, YOLOV7, YOLOV6, YOLOV5, YOLOV4Scaled, YOLOV4, YOLOv3', PPYOLOE, YOLOR, YOLOX ).
-
SaaSHub
SaaSHub - Software Alternatives and Reviews. SaaSHub helps you find the best software and product alternatives
yolor reviews and mentions
-
Explicit and Implicit Knowledge in Object Detection (YOLOR, YOLOv7)
Fellow redditors, can you please explain to me how aforementioned structures work and applied in code? I tried to read carefully the papers on YOLOv7 and YOLOR (https://arxiv.org/pdf/2207.02696.pdf, https://arxiv.org/pdf/2105.04206.pdf) but for me it feels like explanations in text have literally no relation to implementation code (I am totally not into Torch so it makes understanding even harder) (https://github.com/WongKinYiu/yolor/blob/main/utils/layers.py)
-
DeepSort with PyTorch(support yolo series)
WongKinYiu/yolor
-
Build Custom Functions for YOLOv4 with TensorFlow, TFLite & TensorRT
Is there a reason to use YOLOv4 over YOLOv5 or YOLOR?
-
Docker for Absolute Beginners.
I am interested in using Docker for Deep learning models use. On Github people recommend Docker environment to use the model. I am sharing the link to the Github repo. My question is how I can use this GitHub repo and create a docker container
-
[Project]Vehicle Counting + Speed Calculation using YOLOR+ DeepSORT OpenCV Python
So there is a paper on YOLOR by Wong Kin Yiu https://github.com/WongKinYiu/yolor
-
YOLOR (Scaled-YOLOv4-based): The best speed/accuracy ratio for Waymo autonomous driving challenge
[CVPR'21 WAD] Challenge - Waymo Open Dataset: https://waymo.com/open/challenges/2021/real-time-2d-prediction/ YOLOR (Scaled-YOLOv4-based) has the best speed/accuracy ratio on Waymo autonomous driving challenge ((Waymo Open Dataset): Real-time 2D Detection. Thanks Chien-Yao Wang from Academia Sinica and DiDi MapVision team to push Scaled-YOLOv4 further! * DIDI MapVision: https://arxiv.org/abs/2106.08713 * YOLOR https://arxiv.org/abs/2105.04206 * YOLOR-code (Pytorch): https://github.com/WongKinYiu/yolor * Scaled-YOLOv4(CVPR21): https://openaccess.thecvf.com/content/CVPR2021/html/Wang\_Scaled-YOLOv4\_Scaling\_Cross\_Stage\_Partial\_Network\_CVPR\_2021\_paper.html * Scaled-YOLOv4-code (Pytorch): https://github.com/WongKinYiu/ScaledYOLOv4 * YOLOv4: https://arxiv.org/abs/2004.10934 * YOLOv4-code (Darknet, Pytorch, TensorFlow, TRT, OpenCV…): https://github.com/AlexeyAB/darknet#yolo-v4-in-other-frameworks
The DiDi MapVision team has shown excellent results with the YOLOR and DIDI MapVision models, both based on Scaled-YOLOv4: * DIDI MapVision: https://arxiv.org/abs/2106.08713 * YOLOR https://arxiv.org/abs/2105.04206 * YOLOR-code (Pytorch): https://github.com/WongKinYiu/yolor * Scaled-YOLOv4(CVPR21): https://openaccess.thecvf.com/content/CVPR2021/html/Wang_Scaled-YOLOv4_Scaling_Cross_Stage_Partial_Network_CVPR_2021_paper.html * Scaled-YOLOv4-code (Pytorch): https://github.com/WongKinYiu/ScaledYOLOv4 * YOLOv4: https://arxiv.org/abs/2004.10934 * YOLOv4-code (Darknet, Pytorch, TensorFlow, TRT, OpenCV...): https://github.com/AlexeyAB/darknet#yolo-v4-in-other-frameworks
-
[P] YOLOR (Scaled-YOLOv4-based): The best speed/accuracy ratio for Waymo autonomous driving challenge
* YOLOR-code (Pytorch): https://github.com/WongKinYiu/yolor
-
A note from our sponsor - SaaSHub
www.saashub.com | 10 May 2024
Stats
WongKinYiu/yolor is an open source project licensed under GNU General Public License v3.0 only which is an OSI approved license.
yolor is marked as "self-hosted". This means that it can be used as a standalone application on its own.
The primary programming language of yolor is Python.
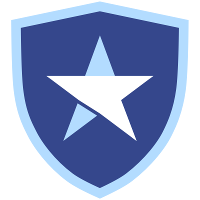
Sponsored