PD-Diffusion Alternatives
Similar projects and alternatives to PD-Diffusion
-
fastdup
fastdup is a powerful free tool designed to rapidly extract valuable insights from your image & video datasets. Assisting you to increase your dataset images & labels quality and reduce your data operations costs at an unparalleled scale.
-
InfluxDB
Power Real-Time Data Analytics at Scale. Get real-time insights from all types of time series data with InfluxDB. Ingest, query, and analyze billions of data points in real-time with unbounded cardinality.
PD-Diffusion reviews and mentions
-
What Does Copyright Say about Generative Models?
>But how much of a song or a painting can you reproduce?
The reason why fair use is vague is specifically to confuse people who ask these kinds of questions. The Supreme Court needed a tool that artists could use to legally smack down people who republish fragments of other people's work, but didn't want to abolish the 1st Amendment in the process. So basically judges have the final say as to whether or not something is novel creativity or in debt to the original. Any hard-and-fast rule beyond "binding precedent applies" is effectively copyright abolition by degrees.
>We lost most of Elizabethan theater because there was no copyright. [..] Without some kind of protection, authors had no interest in publishing at all, let alone publishing accurate texts.
This is a dated example, if only because creative works leave a lot more evidence now than they used to. People today will act to preserve art against the artists own wishes and at great personal risk.
>and it’s easy to suspect that the actual payments will be similar to the royalties musicians get from streaming services: microcents per use
Given the amount of data these systems need (read: more than humanity can provide) I'd say microcents is arguably too high. Remember that you can't actually derive a clear chain of value between one particular training set entry and one particular execution of the model. It's all chucked into a blender that runs on almost-linear algebra and calculus. At best you can detect if parts of the image resemble specific training set examples[0] and pay people slightly more if the model regurgitates training set data.
Let's also keep in mind that a good chunk of the licensing system is based on being able to say no to specific users, or write very tailor-made licensing agreements for specific works or conditions. That's still going to be threatened, even if we can pay sub-Spotify-tier royalties every time a model trains itself on your work.
>It is easy to imagine an AI system that has been trained on the (many) Open Source and Creative Commons licenses.
Working on it: https://github.com/kmeisthax/PD-Diffusion
The thing is, we already have a good database of reusable, public-domain, no-attribution-necessary images; it's called Wikimedia Commons. I really can't fathom why OpenAI didn't start there, other than just an assumption that they were entitled to larger datasets or a feeling that they could get established before anyone sued.
Even then, OpenAI already tried this with computer code and they're getting sued for it anyway, because they never bothered with attribution in the case of training set regurgitation.
[0] This is possible because part of the prompt guidance process involves a thing called CLIP which can do both image and text classification in the same coordinate system.
-
Laion-5B: A New Era of Open Large-Scale Multi-Modal Datasets
The positive solution is to scrape Wikimedia Commons for everything in "Category: PD-Art-old-100" and train from scratch on that data. Wikimedia Commons is well-moderated, the image data is public domain[0], and the labels can be filtered down to CC-BY or CC-BY-SA subsets[1]. Your resulting model will be CC-BY-SA licensed and the output completely copyright-free.
For the record, that's what I've been trying to do[2]; my stumbling blocks have been training time and a bug where my resulting pipeline seems to do the opposite of what I ask[3]. I'm assuming it's because my wikitext parser was broken and CLIP didn't have enough text data to train on; I'll have the answer tomorrow when I have a fully-trained U-Net to play with.
If I can ever get this working, I want to also build a CLIP pipeline that can attribute generated images against the training set. This would make it possible to safely use CC-BY and CC-BY-SA datasets: after generating
[0] At least in the US. Other jurisdictions think that scanning an image recopyrights it, see https://en.wikipedia.org/wiki/National_Portrait_Gallery_and_...
[1] Watch out for anything tagged with https://commons.wikimedia.org/wiki/Template:Royal_Museums_Gr... as that will taint your model.
[2] https://github.com/kmeisthax/PD-Diffusion
[3] https://pooper.fantranslation.org/@kmeisthax/109486435508334...
Stats
kmeisthax/PD-Diffusion is an open source project licensed under Apache License 2.0 which is an OSI approved license.
The primary programming language of PD-Diffusion is Python.
Popular Comparisons
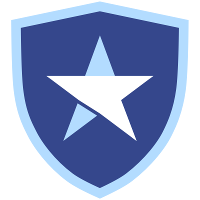
Sponsored