The APIs are flexible and easy-to-use, supporting authentication, user identity, and complex enterprise features like SSO and SCIM provisioning. Learn more →
Top 23 Scala Big Data Projects
-
WorkOS
The modern identity platform for B2B SaaS. The APIs are flexible and easy-to-use, supporting authentication, user identity, and complex enterprise features like SSO and SCIM provisioning.
-
delta
An open-source storage framework that enables building a Lakehouse architecture with compute engines including Spark, PrestoDB, Flink, Trino, and Hive and APIs (by delta-io)
-
InfluxDB
Power Real-Time Data Analytics at Scale. Get real-time insights from all types of time series data with InfluxDB. Ingest, query, and analyze billions of data points in real-time with unbounded cardinality.
-
Reactive-kafka
Alpakka Kafka connector - Alpakka is a Reactive Enterprise Integration library for Java and Scala, based on Reactive Streams and Akka.
-
adam
ADAM is a genomics analysis platform with specialized file formats built using Apache Avro, Apache Spark, and Apache Parquet. Apache 2 licensed.
-
nussknacker
Low-code tool for automating actions on real time data | Stream processing for the users.
-
qbeast-spark
Qbeast-spark: DataSource enabling multi-dimensional indexing and efficient data sampling. Big Data, free from the unnecessary!
-
Clustering4Ever
C4E, a JVM friendly library written in Scala for both local and distributed (Spark) Clustering.
-
Schemer
Schema registry for CSV, TSV, JSON, AVRO and Parquet schema. Supports schema inference and GraphQL API.
-
SaaSHub
SaaSHub - Software Alternatives and Reviews. SaaSHub helps you find the best software and product alternatives
Delta is pretty great, let's you do upserts into tables in DataBricks much easier than without it.
I think the website is here: https://delta.io
Scala Big Data related posts
- Azure data lake - Data Share
- The "Big Three's" Data Storage Offerings
- Medallion/lakehouse architecture data modelling
- How to build a data pipeline using Delta Lake
- whenNotMatchedBySourceUpdate not existing? Trying to upsert parquet into Delta table
- Delta.io/deltalake self hosting
- Delta.io/deltalake self hosting
-
A note from our sponsor - WorkOS
workos.com | 26 Apr 2024
Index
What are some of the best open-source Big Data projects in Scala? This list will help you:
Project | Stars | |
---|---|---|
1 | Apache Spark | 38,320 |
2 | kafka-manager | 11,670 |
3 | delta | 6,897 |
4 | SynapseML | 4,967 |
5 | Scalding | 3,470 |
6 | Scio | 2,520 |
7 | Jupyter Scala | 1,562 |
8 | Reactive-kafka | 1,418 |
9 | adam | 967 |
10 | H2O | 952 |
11 | BIDMach | 913 |
12 | Gearpump | 765 |
13 | Vegas | 729 |
14 | spark-rapids | 720 |
15 | delta-sharing | 674 |
16 | nussknacker | 609 |
17 | metorikku | 576 |
18 | Sparkta | 524 |
19 | Scoobi | 482 |
20 | qbeast-spark | 190 |
21 | Clustering4Ever | 128 |
22 | Schemer | 112 |
23 | Scoozie | 82 |
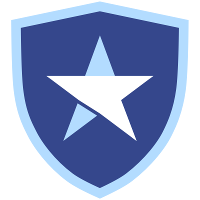
Sponsored