SaaSHub helps you find the best software and product alternatives Learn more →
Top 15 neural-ode Open-Source Projects
-
SciMLBook
Parallel Computing and Scientific Machine Learning (SciML): Methods and Applications (MIT 18.337J/6.338J)
-
torchdyn
A PyTorch library entirely dedicated to neural differential equations, implicit models and related numerical methods
-
InfluxDB
Power Real-Time Data Analytics at Scale. Get real-time insights from all types of time series data with InfluxDB. Ingest, query, and analyze billions of data points in real-time with unbounded cardinality.
-
DiffEqFlux.jl
Pre-built implicit layer architectures with O(1) backprop, GPUs, and stiff+non-stiff DE solvers, demonstrating scientific machine learning (SciML) and physics-informed machine learning methods
-
SciMLTutorials.jl
Tutorials for doing scientific machine learning (SciML) and high-performance differential equation solving with open source software.
-
SciMLSensitivity.jl
A component of the DiffEq ecosystem for enabling sensitivity analysis for scientific machine learning (SciML). Optimize-then-discretize, discretize-then-optimize, adjoint methods, and more for ODEs, SDEs, DDEs, DAEs, etc.
-
SaaSHub
SaaSHub - Software Alternatives and Reviews. SaaSHub helps you find the best software and product alternatives
-
DiffEqBase.jl
The lightweight Base library for shared types and functionality for defining differential equation and scientific machine learning (SciML) problems
-
SciMLBenchmarks.jl
Scientific machine learning (SciML) benchmarks, AI for science, and (differential) equation solvers. Covers Julia, Python (PyTorch, Jax), MATLAB, R
-
DiffEqGPU.jl
GPU-acceleration routines for DifferentialEquations.jl and the broader SciML scientific machine learning ecosystem
-
finite-element-networks
Reference implementation of Finite Element Networks as proposed in "Learning the Dynamics of Physical Systems from Sparse Observations with Finite Element Networks" at ICLR 2022
-
SaaSHub
SaaSHub - Software Alternatives and Reviews. SaaSHub helps you find the best software and product alternatives
Project mention: LNNs - Liquid Neural Networks: Seeking general advice, papers, implementations | /r/datascience | 2023-07-10And here's the repo: https://github.com/raminmh/CfC
Indeed, and this year we created a system for compiling ODE code not just optimized CUDA kernels but also OneAPI kernels, AMD GPU kernels, and Metal. Peer reviewed version is here (https://www.sciencedirect.com/science/article/abs/pii/S00457...), open access is here (https://arxiv.org/abs/2304.06835), and the open source code is at https://github.com/SciML/DiffEqGPU.jl. The key that the paper describes is that in this case kernel generation is about 20x-100x faster than PyTorch and Jax (see the Jax compilation in multiple ways in this notebook https://colab.research.google.com/drive/1d7G-O5JX31lHbg7jTzz..., extra overhead though from calling Julia from Python but still shows a 10x).
The point really is that while deep learning libraries are amazing, at the end of the day they are DSL and really pull towards one specific way of computing and parallelization. It turns out that way of parallelizing is good for deep learning, but not for all things you may want to accelerate. Sometimes (i.e. cases that aren't dominated by large linear algebra) building problem-specific kernels is a major win, and it's over-extrapolating to see ML frameworks do well with GPUs and think that's the only thing that's required. There are many ways to parallelize a code, ML libraries hardcode a very specific way, and it's good for what they are used for but not every problem that can arise.
neural-ode related posts
-
Machine learning and black box numerical solver[D]
-
Accurate and Efficient Physics-Informed Learning Through Differentiable Simulation - Chris Rackauckas (ASA Statistical Computing & Graphics Sections)
-
Why Fortran is easy to learn
-
Composability in Julia: Implementing Deep Equilibrium Models via Neural Odes
-
[R] New directions in Neural Differential Equations
-
JuliaSim - Simulating Reality (new product by Julia Computing)
-
Old programming language is suddenly getting more popular again
-
A note from our sponsor - SaaSHub
www.saashub.com | 14 May 2024
Index
What are some of the best open-source neural-ode projects? This list will help you:
Project | Stars | |
---|---|---|
1 | SciMLBook | 1,796 |
2 | torchdyn | 1,283 |
3 | DiffEqFlux.jl | 839 |
4 | CfC | 796 |
5 | SciMLTutorials.jl | 709 |
6 | SciMLSensitivity.jl | 316 |
7 | 18S096SciML | 303 |
8 | DiffEqBase.jl | 298 |
9 | SciMLBenchmarks.jl | 292 |
10 | ComponentArrays.jl | 277 |
11 | DiffEqGPU.jl | 267 |
12 | torchode | 192 |
13 | finite-element-networks | 60 |
14 | BoundaryValueDiffEq.jl | 40 |
15 | LT-OCF | 20 |
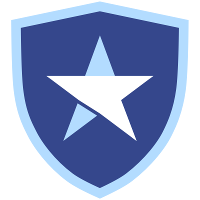
Sponsored