- augmented-interpretable-models VS language-planner
- augmented-interpretable-models VS shap
- augmented-interpretable-models VS scikit-learn-ts
- augmented-interpretable-models VS DeepLearning
- augmented-interpretable-models VS handson-ml
- augmented-interpretable-models VS gan-vae-pretrained-pytorch
- augmented-interpretable-models VS AutoCog
- augmented-interpretable-models VS imodels
- augmented-interpretable-models VS align-transformers
Augmented-interpretable-models Alternatives
Similar projects and alternatives to augmented-interpretable-models
-
language-planner
Official Code for "Language Models as Zero-Shot Planners: Extracting Actionable Knowledge for Embodied Agents"
-
shap
Discontinued A game theoretic approach to explain the output of any machine learning model. [Moved to: https://github.com/shap/shap] (by slundberg)
-
InfluxDB
Power Real-Time Data Analytics at Scale. Get real-time insights from all types of time series data with InfluxDB. Ingest, query, and analyze billions of data points in real-time with unbounded cardinality.
-
scikit-learn-ts
Powerful machine learning library for Node.js – uses Python's scikit-learn under the hood.
-
WorkOS
The modern identity platform for B2B SaaS. The APIs are flexible and easy-to-use, supporting authentication, user identity, and complex enterprise features like SSO and SCIM provisioning.
-
imodels
Interpretable ML package 🔍 for concise, transparent, and accurate predictive modeling (sklearn-compatible).
augmented-interpretable-models reviews and mentions
-
[R] Emb-GAM: an Interpretable and Efficient Predictor using Pre-trained Language Models
Deep learning models have achieved impressive prediction performance but often sacrifice interpretability, a critical consideration in high-stakes domains such as healthcare or policymaking. In contrast, generalized additive models (GAMs) can maintain interpretability but often suffer from poor prediction performance due to their inability to effectively capture feature interactions. In this work, we aim to bridge this gap by using pre-trained neural language models to extract embeddings for each input before learning a linear model in the embedding space. The final model (which we call Emb-GAM) is a transparent, linear function of its input features and feature interactions. Leveraging the language model allows Emb-GAM to learn far fewer linear coefficients, model larger interactions, and generalize well to novel inputs (e.g. unseen ngrams in text). Across a variety of NLP datasets, Emb-GAM achieves strong prediction performance without sacrificing interpretability. All code is made available on Github.
Stats
microsoft/augmented-interpretable-models is an open source project licensed under MIT License which is an OSI approved license.
The primary programming language of augmented-interpretable-models is Jupyter Notebook.
Popular Comparisons
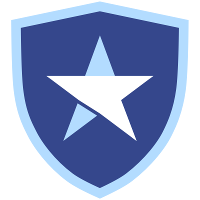
Sponsored