zep-js
continuous-eval
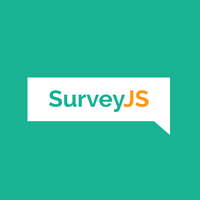
zep-js | continuous-eval | |
---|---|---|
3 | 4 | |
19 | 342 | |
- | 9.1% | |
9.3 | 8.7 | |
3 days ago | 13 days ago | |
TypeScript | Python | |
Apache License 2.0 | Apache License 2.0 |
Stars - the number of stars that a project has on GitHub. Growth - month over month growth in stars.
Activity is a relative number indicating how actively a project is being developed. Recent commits have higher weight than older ones.
For example, an activity of 9.0 indicates that a project is amongst the top 10% of the most actively developed projects that we are tracking.
zep-js
-
Show HN: Ellipsis – Automated PR reviews and bug fixes
Hmm, that searches issues, which isn't the best way to see Ellipsis' work.
Example of PR review: https://github.com/getzep/zep-js/pull/67#discussion_r1594781...
Example of issue-to-PR: https://github.com/getzep/zep/issues/316
Example of bug fix on a PR: https://github.com/jxnl/instructor/pull/546#discussion_r1544...
-
How do domain-specific chatbots work? A retrieval augmented generation overview
Relatedly, to have a useful chatbot you need to track chat history in a way very similar to augmenting with document retrieval, but you may need to generate embeddings and summaries as you go.
A friend of mine is working on an OSS memory system for chat apps that helps store, retrieve, summarize chat history, and documents to now, I believe, on top of LangChain: https://www.getzep.com/
-
Show HN: Zep – Long-Term Memory Store for Conversational AI Apps
- When storing messages long-term, developers are exposed to privacy and regulatory obligations around PII, retention, and deletion of user data.
Zep aims to solve these challenges.
Zep and its Python and [Ja](https://github.com/getzep/zep-js)vascript client libraries have been open-sourced under the Apache License.
Learn more and contribute:
continuous-eval
-
Show HN: Ellipsis – Automated PR reviews and bug fixes
Hi HN, hunterbrooks and nbrad here from Ellipsis (https://www.ellipsis.dev). Ellipsis automatically reviews your PRs when opened and on each new commit. If you tag @ellipsis-dev in a comment, it can make changes to the PR (via direct commit or side PR) and answer questions, just like a human.
Demo video: https://www.youtube.com/watch?v=X61NGZpaNQA
So far, we have dozens of open source projects and companies using Ellipsis. We seem to have landed in a kind of sweet spot where there’s a good match between the current capabilities of AI tools and the actual needs of software engineers - this doesn’t replace human review, but it saves you time by catching/fixing lots of small silly stuff.
Here’s an example in the wild: https://github.com/relari-ai/continuous-eval/pull/38, where Ellipsis (1) adds a PR summary; (2) finds a bug and adds a review comment; (3) after a [human] user comments, generates a side PR with the fix; and (4) after a (human) user merges the side PR and adds another commit, re-reviews the PR and approves it
Here’s another example: https://github.com/SciPhi-AI/R2R/pull/350#pullrequestreview-..., where Ellipsis adds several comments with inline suggestions that were directly merged by the developer.
You can configure Ellipsis in natural language to enforce custom rules, style guides, or conventions. For example, here’s how the `jxnl/instructor` repo uses natural language rules to make sure that docs are kept in sync: https://github.com/jxnl/instructor/blob/main/ellipsis.yaml#L..., and here’s an example PR that Ellipsis came up with based on those rules: https://github.com/jxnl/instructor/pull/346.
Don’t worry, your code is never stored or used to train models (https://docs.ellipsis.dev/security).
Installing into your repo takes 2 clicks at https://www.ellipsis.dev. We’d really appreciate your feedback, thoughts, and ideas!
-
Launch HN: Relari (YC W24) – Identify the root cause of problems in LLM apps
Hi HN, we are the founders of Relari, the company behind continuous-eval (https://github.com/relari-ai/continuous-eval), an evaluation framework that lets you test your GenAI systems at the component level, pinpointing issues where they originate.
We experienced the need for this when we were building a copilot for bankers. Our RAG pipeline blew up in complexity as we added components: a query classifier (to triage user intent), multiple retrievers (to grab information from different sources), filtering LLM (to rerank / compress context), a calculator agent (to call financial functions) and finally the synthesizer LLM that gives the answer. Ensuring reliability became more difficult with each of these we added.
When a bad response was detected by our answer evaluator, we had to backtrack multiple steps to understand which component(s) made a mistake. But this quickly became unscalable beyond a few samples.
I did my Ph.D. in fault detection for autonomous vehicles, and I see a strong parallel between the complexity of autonomous driving software and today's LLM pipelines. In self-driving systems, sensors, perception, prediction, planning, and control modules are all chained together. To ensure system-level safety, we use granular metrics to measure the performance of each module individually. When the vehicle makes an unexpected decision, we use these metrics to pinpoint the problem to a specific component. Only then we can make targeted improvements, systematically.
Based on this thinking, we developed the first version of continuous-eval for ourselves. Since then we’ve made it more flexible to fit various types of GenAI pipelines. Continuous-eval allows you to describe (programmatically) your pipeline and modules, and select metrics for each module. We developed 30+ metrics to cover retrieval, text generation, code generation, classification, agent tool use, etc. We now have a number of companies using us to test complex pipelines like finance copilots, enterprise search, coding agents, etc.
As an example, one customer was trying to understand why their RAG system did poorly on trend analysis queries. Through continuous-eval, they realized that the “retriever” component was retrieving 80%+ of all relevant chunks, but the “reranker” component, that filters out “irrelevant” context, was dropping that to below 50%. This enabled them to fix the problem, in their case by skipping the reranker for certain queries.
We’ve also built ensemble metrics that do a surprisingly good job of predicting user feedback. Users often rate LLM-generated answers by giving a thumbs up/down about how good the answer was. We train our custom metrics on this user data, and then use those metrics to generate thumbs up/down ratings on future LLM answers. The results turn out to be 90% aligned with what the users say. This gives developers a feedback loop from production data to offline testing and development. Some customers have found this to be our most unique advantage.
Lastly, to make the most out of evaluation, you should use a diverse dataset—ideally with ground truth labels for comprehensive and consistent assessment. Because ground truth labels are costly and time-consuming to curate manually, we also have a synthetic data generation pipeline that allows you to get started quickly. Try it here (https://www.relari.ai/#synthetic_data_demo)
What’s been your experience testing and iterating LLM apps? Please let us know your thoughts and feedback on our approaches (modular framework, leveraging user feedback, testing with synthetic data).
- Show HN: Ellipsis – Automatic pull request reviews
- Show HN: Granular Evaluation of GenAI Pipelines
What are some alternatives?
zep - Zep: Long-Term Memory for AI Assistants.
text-to-image-eval - Evaluate custom and HuggingFace text-to-image/zero-shot-image-classification models like CLIP, SigLIP, DFN5B, and EVA-CLIP. Metrics include Zero-shot accuracy, Linear Probe, Image retrieval, and KNN accuracy.
zep-python - Zep: Long-Term Memory for AI Assistants (Python Client)
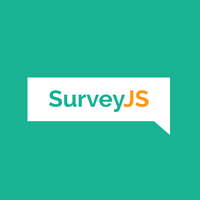